Jeffrey L. Krichmar
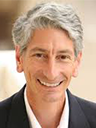
Session: F. Informatics IV
Will talk about: Towards a modeling framework for the efficient creation, simulation and analysis of brain functions
Jeffrey L. Krichmar received a B.S. in Computer Science in 1983 from the University of Massachusetts at Amherst, a M.S. in Computer Science from The George Washington University in 1991, and a Ph.D. in Computational Sciences and Informatics from George Mason University in 1997. He spent 15 years as a software engineer on projects ranging from the PATRIOT Missile System at the Raytheon Corporation to Air Traffic Control for the Federal Systems Division of IBM. In 1997, he became an assistant professor at The Krasnow Institute for Advanced Study at George Mason University. From 1999 to 2007, he was a Senior Fellow in Theoretical Neurobiology at The Neurosciences Institute. Currently, he is a Professor at the University of California, Irvine, in the Department of Cognitive Sciences, with a joint appointment in the Department of Computer Science. He leads the Cognitive Anteater Robotics Laboratory (CARL) at UC Irvine where they test computational models of nervous system function on robotic platforms and large-scale, highly detailed models of neuronal circuits. He has been involved in these fields, which are known as neuromorphic engineering and neurorobotics, for over 15 years. He has published nearly 100 articles and holds seven patents.
Key goals for computational neuroscience and neuroinformatics are to develop innovative tools and technologies to help understand the human brain. To reach this understanding, we need simulations that can efficiently integrate information across the nervous system’s large temporal and spatial scales. Moreover, for these simulations to have explanatory power, they must be accompanied with analysis tools, and they need to provide a linkage to experimental data at several levels. To that end, we have developed a simulation framework, called CARLsim, to specify large-scale brain spiking neural networks (SNNs) having neurobiological details at the neuron and synaptic level. CARLsim is an efficient, easy-to-use, GPU-accelerated framework for simulating SNNs on the order of hundreds of thousands to millions of neurons in near real-time on desktop computers. CARLsim was originally developed to support the DARPA SyNAPSE project on neuromorphic computing, but now is publicly available as a general-purpose SNN simulation environment. In this talk, I will review how this approach has been used to implement a broad range of computational models that include simulations of visual processing, neuromodulation, synaptic plasticity, and attention. I will also introduce an automated parameter-tuning framework to efficiently search the large parameter spaces of SNNs. Using this framework, our group was able to replicate neurophysiological recordings from the rat during spatial navigation tasks. A SNN that can faithfully simulate empirical data may help to provide further insight into the function of the brain area under study. Furthermore, our approach for replicating electrophysiological data may aid in the future development of neuroprostheses that replace damaged neural tissue and restore lost cognitive function.